In this example we show you how to use a Jupyter Notebook with the ohsome API to analyse mapping efforts for a HOT Tasking Manager project in Nepal!
Analyse HOT Tasking Manager Projects with the ohsome API
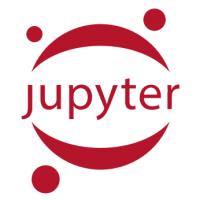
Warning: file_get_contents(): SSL operation failed with code 1. OpenSSL Error messages: error:14090086:SSL routines:ssl3_get_server_certificate:certificate verify failed in /var/www/html/wp-content/plugins/nbconvert/nbconvert.php on line 87
Warning: file_get_contents(): Failed to enable crypto in /var/www/html/wp-content/plugins/nbconvert/nbconvert.php on line 87
Warning: file_get_contents(https://nbviewer.jupyter.org/url/github.com/GIScience/ohsome-examples/blob/master/python/jupyter-notebooks/ohsome_api_hot_tm_project1008.ipynb): failed to open stream: operation failed in /var/www/html/wp-content/plugins/nbconvert/nbconvert.php on line 87
Warning: DOMDocument::loadHTML(): Empty string supplied as input in /var/www/html/wp-content/plugins/nbconvert/nbconvert.php on line 121
Comments are closed.