Volume 26, Issue 8 of Transactions in GIS, published in December 2022, has a cover story and it’s us- well, our article anyway. Besides uniting our love of math, mapping, and Mozambique, we’re hoping to use our moment of fame to explain our proposed solution to the issue of missing mapping data in the Global South. Read on for our inspiration, our method, and even an autograph (if you ask nicely).
Filling in the Blanks
What do you do when you have the tools, the motivation, and the need, but not the data?
In an innovative paper first published in Transactions in GIS in May 2022, a collaborative team of current and former researchers from HeiGIT and GIScience including Hao Li, Benjamin Herfort, Sven Lautenbach, Jiaoyan Chen, and Alexander Zipf apply a novel few-shot transfer learning method (FSTL) to the problem of improving humanitarian mapping workflows in the Global South.
Despite the recent much-needed leaps forward in data availability in the developing world from humanitarian mapping campaigns, many large rural areas remain completely unmapped, hindering disaster routing and other disaster preparation and response efforts. The criticality of such routing, preparation, and response efforts can be seen from our work with last year’s floods in Pakistan, the global coverage of our Open Healthcare Access map, and our publication assessing the damage done by Cyclone Idai.
Our team’s bid to tackle the speed and efficiency concerns for these workflows is proposing the FSTL method to increase the accuracy of OSM missing building detection. Even with only one training shot, the approach already demonstrated considerable success.
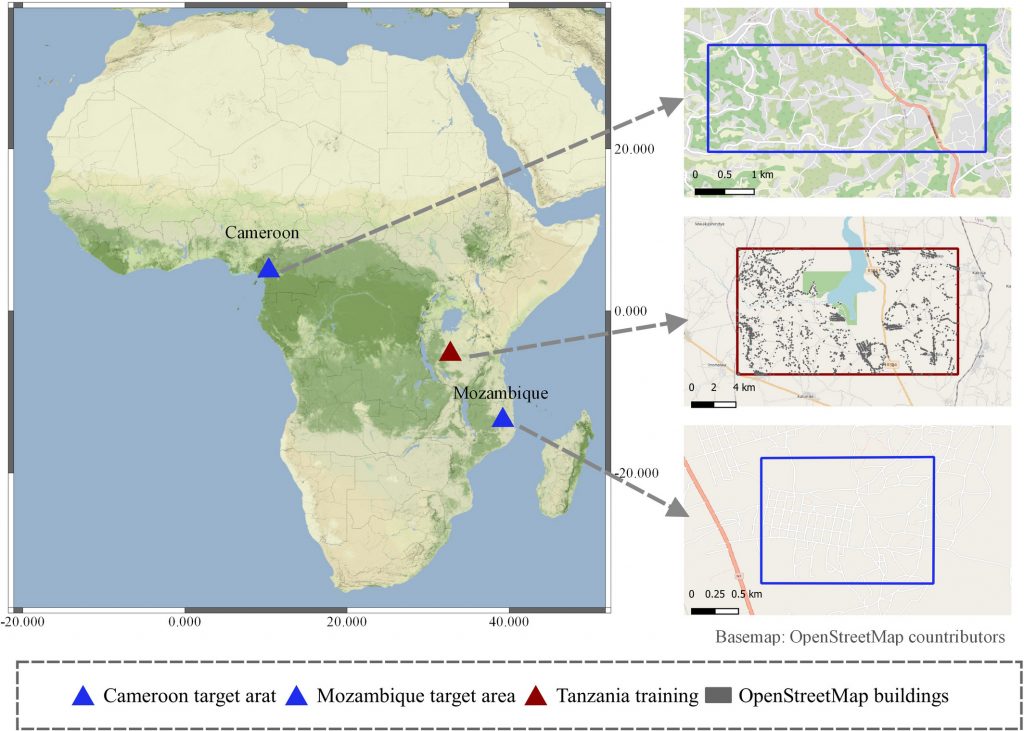
The Nitty-Gritty
The team trained two popular object detection models (Faster R-CNN and SSD) in a training area in Tanzania and transferred the model to target areas in Cameroon and Mozambique. The FSTL method significantly improved the base model performance even with only one training shot.
They also successfully produced a grid-based OSM missing building map (DeepVGI) of 10 m spatial resolution with over 96% overall accuracy (ACC) and 0.85 Matthews correlation coefficient (MCC) in both Cameroon and Mozambique. Such maps show great potential to assess and estimate the overall completeness of OSM buildings to support humanitarian mapping activities, especially in places where other (e.g., buildings, roads) datasets are not available.
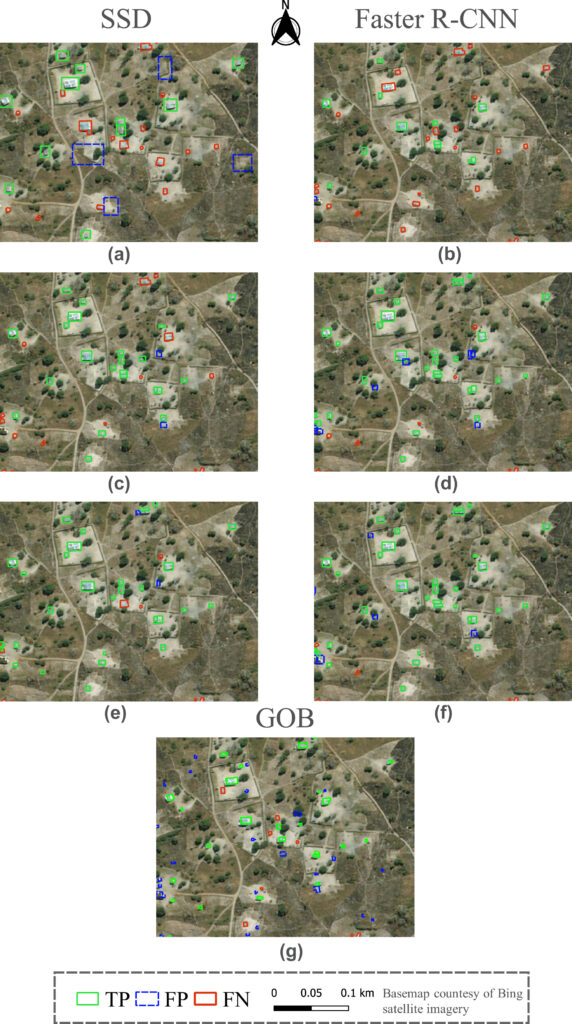
OSM missing building maps (DeepVGI) produced by the proposed FSTL method showed superior mapping accuracies in both target areas. The proposed FSTL method required only limited training shots (e.g., only one shot) to achieve a fast and remarkable accuracy improvement. The proposed FSTL method of reusing a building detection model trained on remote areas provides a promising solution to existing challenges of humanitarian mapping.
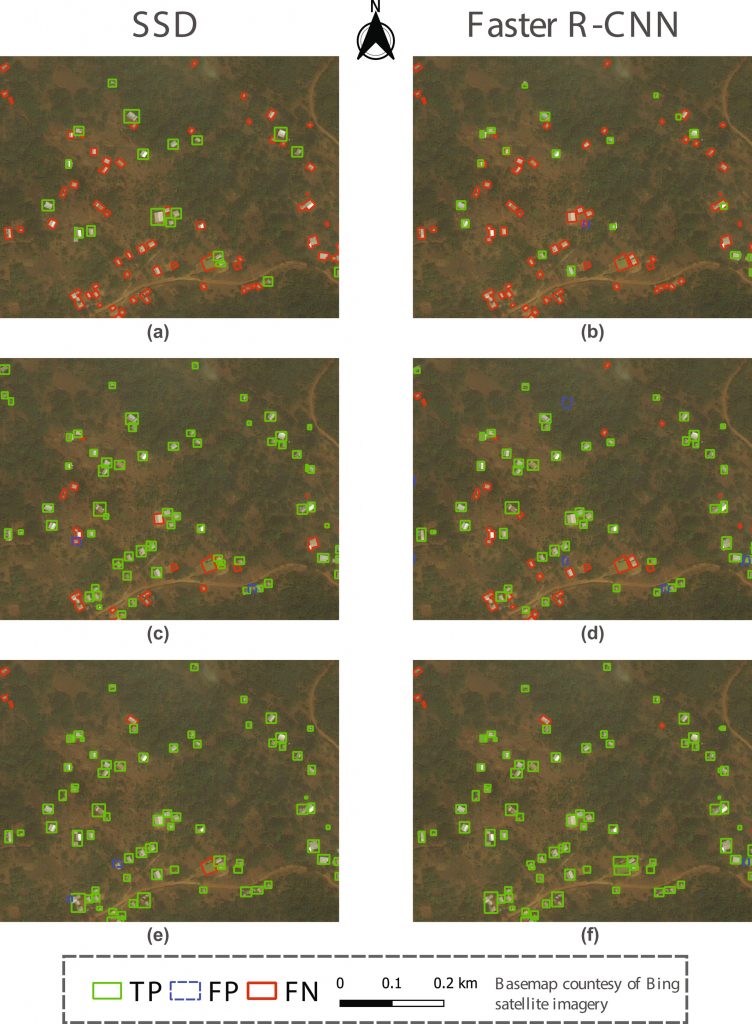
Current Limitations
The team identified three limitations for this case study.
-
The current work focused on areas where OSM buildings were completely missing and future research is needed to validate the proposed method in areas where buildings are partly mapped in OSM. In those partly mapped area, one could directly consider existing OSM buildings as the FSTL training shots and seek to assess the relative completeness of OSM using a similar DeepVGI layer.
-
As the proposed method was implemented and validated only in sub-Saharan African, a global overview of such a method applied in different geographically remote rural areas (e.g., urban contexts, climate, vegetation, settlement types) is still missing.
-
Both the current Faster R-CNN and the SSD model only detect bounding boxes of buildings but not their footprints. Further research is encouraged to extend the FSTL method toward the automatic extraction of building footprints in vector formats, for example, using the Mask R-CNN architecture (He et al., 2017) and with post-processing similar as (Sirko et al., 2021).
Future Research Avenues
To address the aforementioned limitations, future works are encouraged to adopt or extend the FSTL method proposed in this article and further investigate several research directions.
-
Besides assessing the relative completeness, the availability of ML-generated layers (e.g., DeepVGI) opens new opportunities of revisiting classic OSM extrinsic quality assessment methods (Hagenauer & Helbich, 2012; Hecht et al., 2013), which commonly rely on external reference datasets.
-
Thanks to the contribution of volunteers, the large amount of existing finished humanitarian mapping projects worldwide, for instance from MapSwipe App and HOT Tasking Manager (Herfort et al., 2021), provides a great overview of vulnerable rural areas with a pressing need of mapping in OSM. One can think about scaling up the FSTL method to these remote areas and further investigating the impact of different types (or levels) of urban contexts on the model performance.
-
By thinking about how ML methods can best benefit OSM, especially in rural areas, recent discussion in Mooney & Galvan (2021) and Vargas-Muñoz et al. (2020) shared important insights from either remote sensing or OSM community perspectives. As an intuitive application, one can apply the FSTL method to estimate the overall time and effort required to map all OSM missing buildings, then take these factors into consideration when organizing humanitarian mapping campaigns and volunteer contributions.
-
The previous work in Herfort et al. (2019) reported an inspiring finding that advanced ML algorithms can potentially improve the accuracy and accelerate the speed of current humanitarian mapping method using the MapSwipe App. Therefore, another potential application of the FSTL method could be assisting human mappers in rural areas by giving them mapping hints based on machine-generated results, such as an early attempt with the RapiD tool from Facebook (RapiD, 2019).
In this context, the team aimed to emphasize the necessity of machine-assisted mapping with the lessons learned in this article. Our future work will seek to develop intelligent OSM mapping workflows by keeping humans and machines in a loop.
In A Sentence
In conclusion, the lessons learned in this article sheds light on facilitating and accelerating current humanitarian mapping workflow by efficiently reusing existing ML models via few-shot learning and accurately detecting OSM missing buildings in rural sub-Saharan Africa areas.
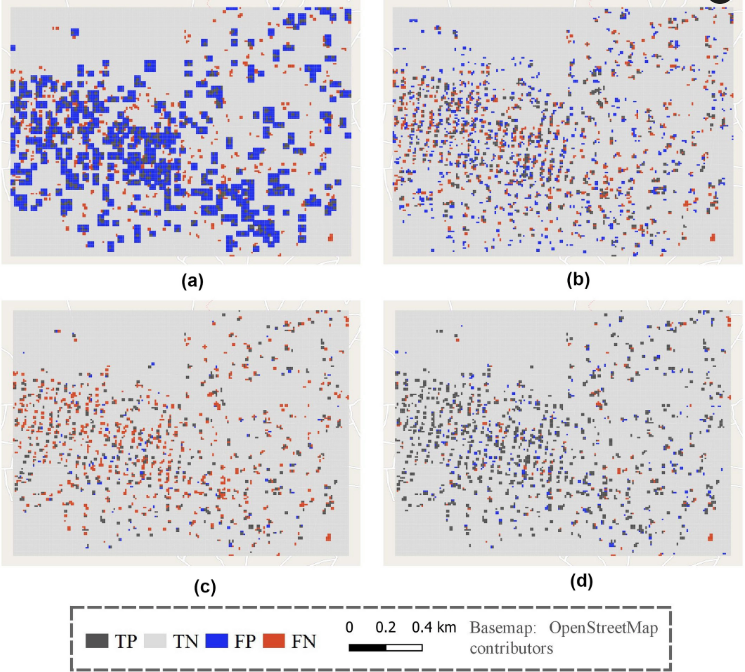
For full paper and all references:
Li, H., Herfort, B., Lautenbach, S., Chen, J. & Zipf, A. (2022). Improving OpenStreetMap missing building detection using few-shot transfer learning in sub-Saharan Africa. Transactions in GIS, 00, 1– 22. https://doi.org/10.1111/tgis.12941
Previous related work:
-
Herfort, B., Lautenbach, S., Porto de Albuquerque, J., Anderson, J., Zipf, A. (2021): The evolution of humanitarian mapping within the OpenStreetMap community. Scientific Reports 11, 3037 (2021).
-
Pisl, J., Li, H., Lautenbach, S., Herfort, B., and Zipf, A. (2021): Detecting OpenStreetMap missing buildings by transferring pre-trained deep neural networks, AGILE GIScience Ser., 2, 39,
AGILE-GISS – Detecting OpenStreetMap missing buildings by transferring pre-trained deep neural networks .
-
Geldsetzer, P.; Reinmuth, M.; O Ouma, P.; Lautenbach, S.; A Okiro, E.; Bärnighausen, T.; Zipf, A. (2020): Mapping physical access to health care for older adults in sub-Saharan Africa and implications for the COVID-19 response: a cross-sectional analysis The LANCET Healthy Longevity, Vol 1, Issue 1. Mapping physical access to health care for older adults in sub-Saharan Africa and implications for the COVID-19 response: a cross-sectional analysis
-
Li, H., Herfort, B., Huang, W., Zia, M., and Zipf, A. (2020): Exploration of OpenStreetMap Missing Built-up Areas using Twitter Hierarchical Clustering and Deep Learning in Mozambique. ISPRS Journal of Photogrammetry and Remote Sensing.
Exploration of OpenStreetMap missing built-up areas using twitter hierarchical clustering and deep learning in Mozambique
-
Herfort, B., Li, H., Fendrich, S., Lautenbach, S., Zipf, A. (2019): Mapping Human Settlements with Higher Accuracy and Less Volunteer Efforts by Combining Crowdsourcing and Deep Learning. Remote Sensing 11(15), 1799. Mapping Human Settlements with Higher Accuracy and Less Volunteer Efforts by Combining Crowdsourcing and Deep Learning
-
Li, H., Herfort, B., Zipf, A. (2019): Estimating OpenStreetMap Missing Built-up Areas using Pre-trained Deep Neural Networks. Proceedings of the 22nd AGILE Conference on Geographic Information Science, Limassol, Cyprus.
-
Zipf, A, Chen, J. (2017): Humanitarian Mapping with Deep Learning and Volunteered Geographic Information. BIG 2017 (BigData Innovators Gathering). Perth. co-located with WWW2017.
-
Chen, J., Y. Zhou, A. Zipf and H. Fan (2018): Deep Learning from Multiple Crowds: A Case Study of Humanitarian Mapping. IEEE Transactions on Geoscience and Remote Sensing (TGRS). 1-10. https://doi.org/10.1109/TGRS.2018.2868748
-
Barron, C., Neis, P. & Zipf, A. (2013): A Comprehensive Framework for Intrinsic OpenStreetMap Quality Analysis. Transactions in GIS, DOI: 10.1111/tgis.12073.
General Overview:
-
Yan, Y., C. Feng, W. Huang, H. Fan, Y. Wang & A. Zipf (2020): Volunteered geographic information research in the first decade: a narrative review of selected journal articles in GIScience. International Journal of Geographical Information Science, DOI: 10.1080/13658816.2020.1730848
-
Degrossi L.C., J. Porto de Albuquerque, R. dos Santos Rocha, A. Zipf (2018): A taxonomy of quality assessment methods for volunteered and crowdsourced geographic information. Transactions in GIS (TGIS). Wiley. DOI:10.1111/tgis.12329.
-
Jokar Arsanjani, J., Zipf, A., Mooney, P., Helbich, M. (Eds.)(2015): OpenStreetMap in GIScience: Experiences, Research, and Applications. Series: Lecture Notes in Geoinformation and Cartography. 2015, VII, 373 p. Sringer Science. Heidelberg, Berlin. ISBN 978-3-319-14279-1