Featured Image: Figure 3 from paper.
One of the contributions showcased by the HeiGIT/GeoScience team in last year’s Free and Open Source Software for Geospatial (FOSS4G) 2022 conference was Moritz Schott, Sven Lautenbach, Leonie Großchen, and Alexander Zipf’s novel paper “OpenStreetMap Element Vectorisation: A Tool for High Resolution Data Insights and its Usability in the Land-use and Land-cover Domain.”
The contribution presents a tool to address the much-discussed issue of fitness of purpose. As researchers and users take advantage of OpenStreetMap’s spatial analysis and location-based services applications, they often turn to intrinsic data analysis due to a lack of extrinsic high-quality datasets. One limitation to this reliance on intrinsic data analysis is the tendency for this information to focus on specific topics or regions.
The new tool, OpenStreetMap Element Vectorisation, currently provides access to 32 attributes or indicators at the level of single OpenStreetMap objects. These indicators cover aspects concerning the element itself, surrounding objects and the editors of the object. A graphic workflow illustrates the steps required to use the tool from file configuration and database setup to result output.
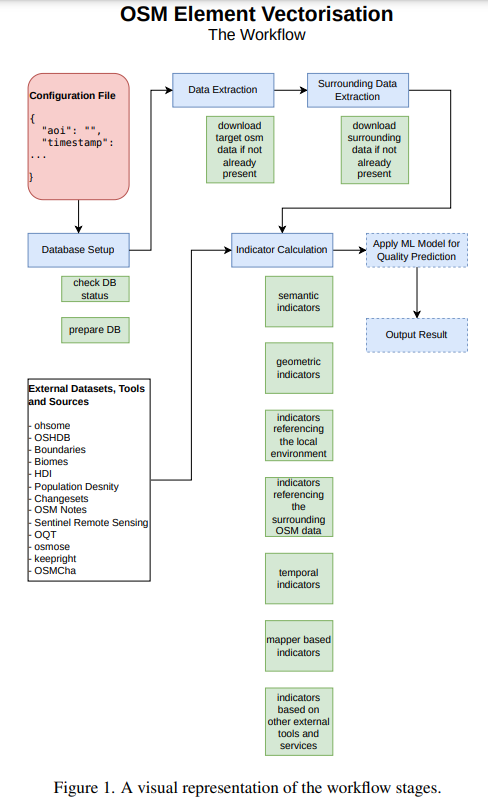
Testing Grounds
The paper not only introduced but sought to test OpenStreetMap Element Vectorisation’s usability for the use case of LULC elements of land-use and land-cover polygons.
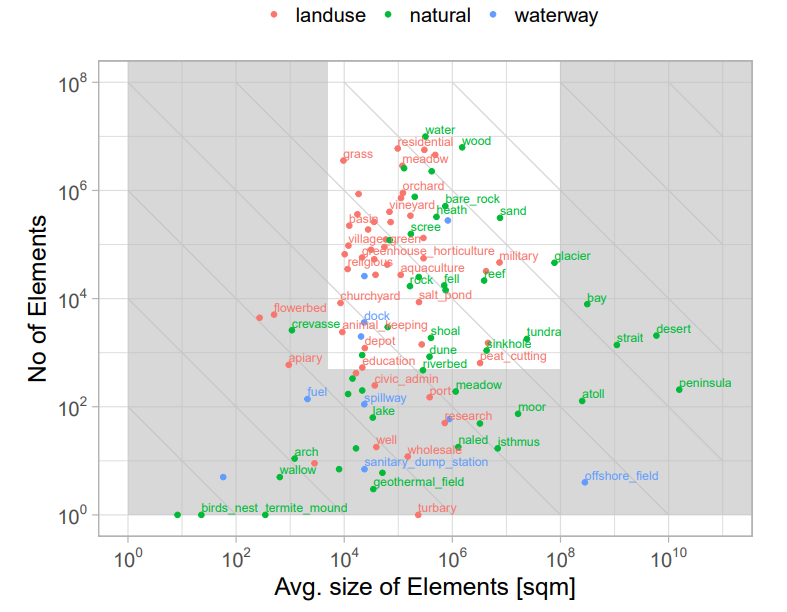
Figure 2 from paper. OSM LULC tags by their frequency and size, based on all OSM LULC polygons in 2021. Colors represent the tag-key. The gray horseshoe defines an area where tags can be classified as too small (too detailed), too large (too general) or too rare (not in use). Diagonal grid lines define tags of equal importance meaning equal global areal coverage
The researchers found that OpenStreetMap objects in more densely-populated areas tended to be smaller, while the age and size of the objects differed across continents. In Europe and North America, the researchers detected older and smaller objects.
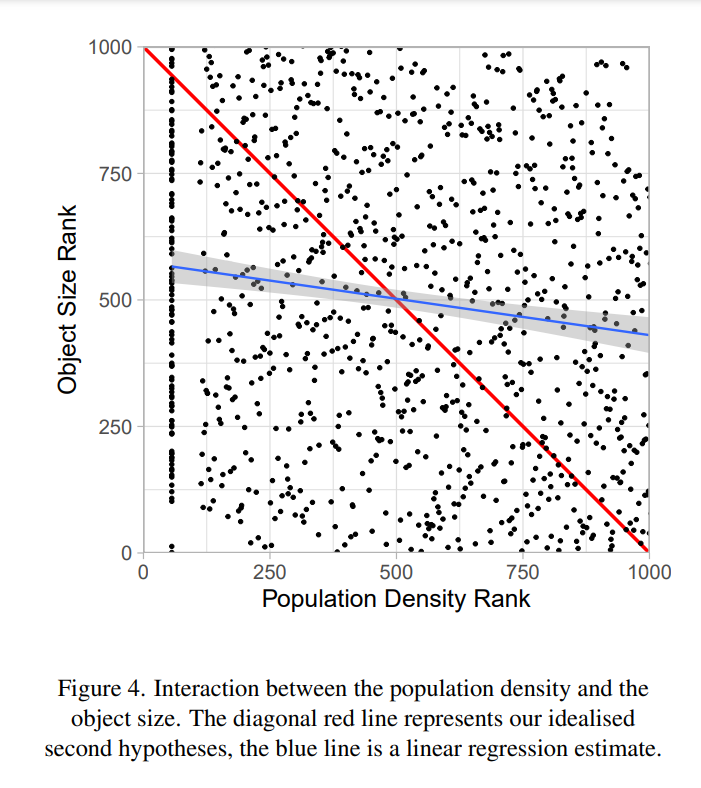
A k-means cluster analysis was used to identify groups in the data, finding a cluster highly influenced by North American lakes that originate from imports.
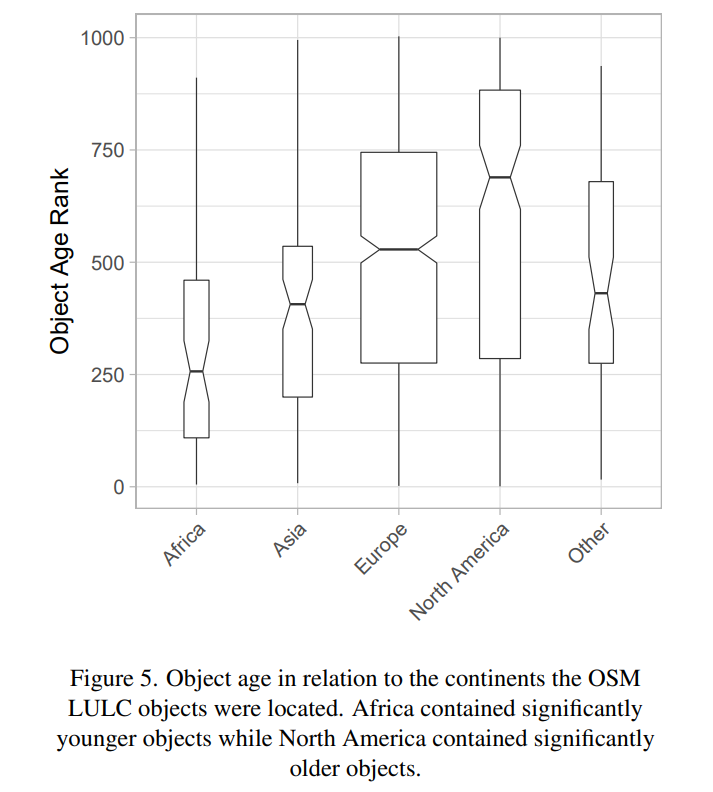
Eye to the Future
The tool offers amble opportunities for future research, supports the OpenStreetMap community by making informed planning decisions for future activities and enables data consumers to make informed decisions on data usage. While the development was made with land-use and land-cover information in mind, the tool can be seamlessly applied to any polygonal OpenStreetMap data and also supports linear and point data.
For full paper and all references:
- Schott, M., Lautenbach, S., Großchen , L., & Zipf, A. (2022). openstreetmap element vectorisation – a tool for high resolution data insights and its usability in the land-use and land-cover domain. The International Archives of the Photogrammetry, Remote Sensing and Spatial Information Sciences, XLVIII-4/W1-2022. https://doi.org/10.5194/isprs-archives-XLVIII-4-W1-2022-395-2022.
Related work:
- Bruckner, J., Schott, M., Zipf, A., Lautenbach, S., 2021. Assessing shop completeness in OpenStreetMap for two federal states in Germany. AGILE: GIScience Series, 2, 20.
- Herfort, B., Lautenbach, S., de Albuquerque, J. P., Anderson, J., Zipf, A., 2021. The evolution of humanitarian mapping within the OpenStreetMap community. Scientific Reports, 11(1), 1–15.
- Jokar Arsanjani, J., Mooney, P., Zipf, A., Schauss, A., 2015. Quality assessment of the contributed land use information from openstreetmap versus authoritative datasets. J. Jokar Arsanjani, A. Zipf, P. Mooney, M. Helbich (eds), OpenStreetMap in GIScience: Experiences, Research, and Applications, Springer International Publishing, Cham, 37–58.
- Neis, P., Zielstra, D., Zipf, A., 2013. Comparison of Volunteered Geographic Information Data Contributions and Community Development for Selected World Regions. Future Internet, 5(2), 282–300.
- Neis, P., Zipf, A., 2012. Analyzing the Contributor Activity of a Volunteered Geographic Information Project — The Case of OpenStreetMap. ISPRS International Journal of GeoInformation, 1(2), 146–165.
- Raifer, M., Troilo, R., Kowatsch, F., Auer, M., Loos, L., Marx, S., Przybill, K., Fendrich, S., Mocnik, F.-B., Zipf, A., 2019. OSHDB: a framework for spatio-temporal analysis of OpenStreetMap history data. Open Geospatial Data, Software and Standards, 4(1), 1–12.
- Schott, M., Grinberger, A. Y., Lautenbach, S., Zipf, A., 2021. The Impact of Community Happenings in OpenStreetMap—Establishing a Framework for Online Community Member Activity Analyses. ISPRS International Journal of GeoInformation, 10(3), 164.
- Schott, M., Zell, A., Lautenbach, S., Zipf, A., Demir, B., 2022. LULC multi-tags based on OSM, Version 0.1. https://gitlab.gistools.geog.uni-heidelberg.de/giscience/idealvgi/osm-multitag.
- Schultz, M., Voss, J., Auer, M., Carter, S., Zipf, A., 2017. Open land cover from OpenStreetMap and remote sensing. International Journal of Applied Earth Observation and Geoinformation, 63, 206-213.
- Zielstra, D., Zipf, A., 2010. A comparative study of proprietary geodata and volunteered geographic information for Germany. 13th AGILE international conference on geographic information science, 2010, 1–15.